
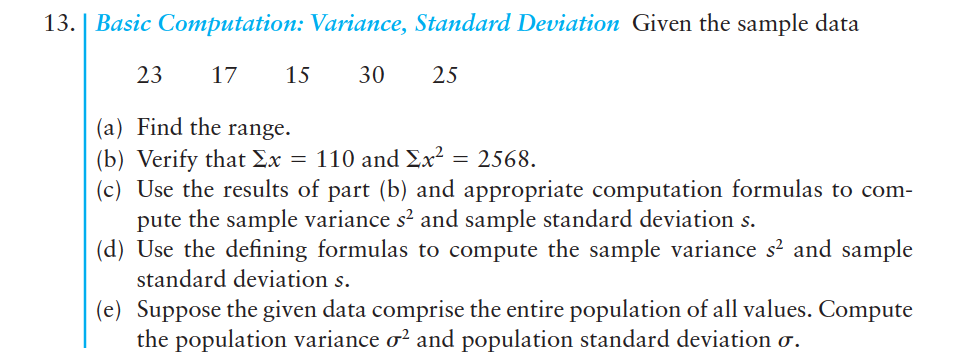
The skew measures how symmetrical the data set is, or whether it has more high values, or more low values. To calculate the standard deviation, take the square root of the variance.
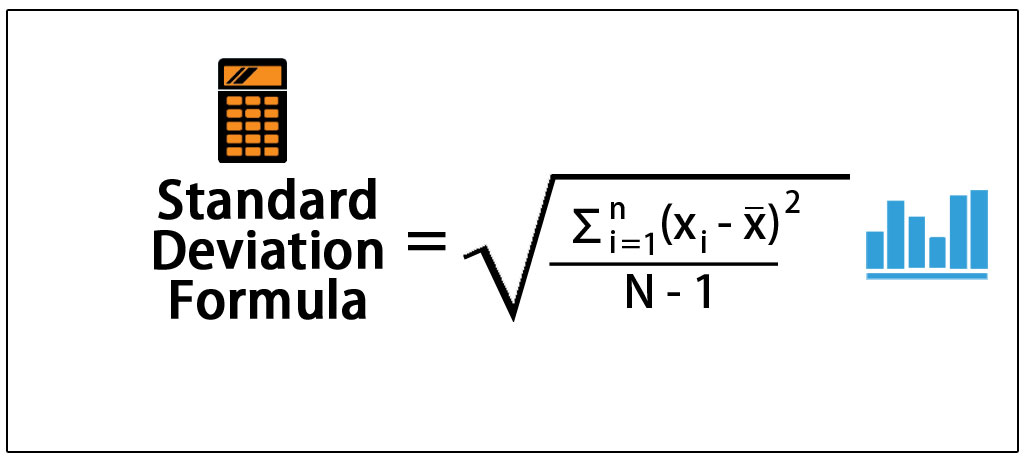
The median is therefore more robust than the mean, because it is not affected by outliers, and grouping is likely to lead to very few changes.Įfficiency is a measure of how well the summary measure uses all the data.Ī more efficient measure uses more data. A robust measure is NOT sensitive to these changes. These changes in data quality can arise either through outliers, extreme values at either end, or from actions taken during analysis, such as grouping the data for further analysis. Robustness is a measure of how sensitive the summary measure is to changes in data quality. There are two constructs (ideas or concepts) that are commonly used to assess summary measures such as mean, median and mode. This might, for example, be ‘men’, ‘women’, and ‘other/no gender specified’, grouped by age categories 20–29, 30–39, 40–49 and 50–59.Īssessing summary measures: robustness and efficiency One of the most common techniques used for summarising is using graphs, particularly bar charts, which show every data point in order, or histograms, which are bar charts grouped into broader categories.Īn example is shown below, which uses three sets of data, grouped by four categories. For example, if you think you may be interested in differences by age, the first thing to do is probably to group your data in age categories, perhaps ten- or five-year chunks. The starting point is usually to group the raw data into categories, and/or to visualise it. The first thing to do with any data is to summarise it, which means to present it in a way that best tells the story. Summarising Data: Grouping and Visualising This page provides a brief summary of some of the most common techniques for summarising your data, and explains when you would use each one. There is a wide range of possible techniques that you can use. It’s now time to carry out some statistical analysis to make sense of, and draw some inferences from, your data. Once you have collected quantitative data, you will have a lot of numbers.

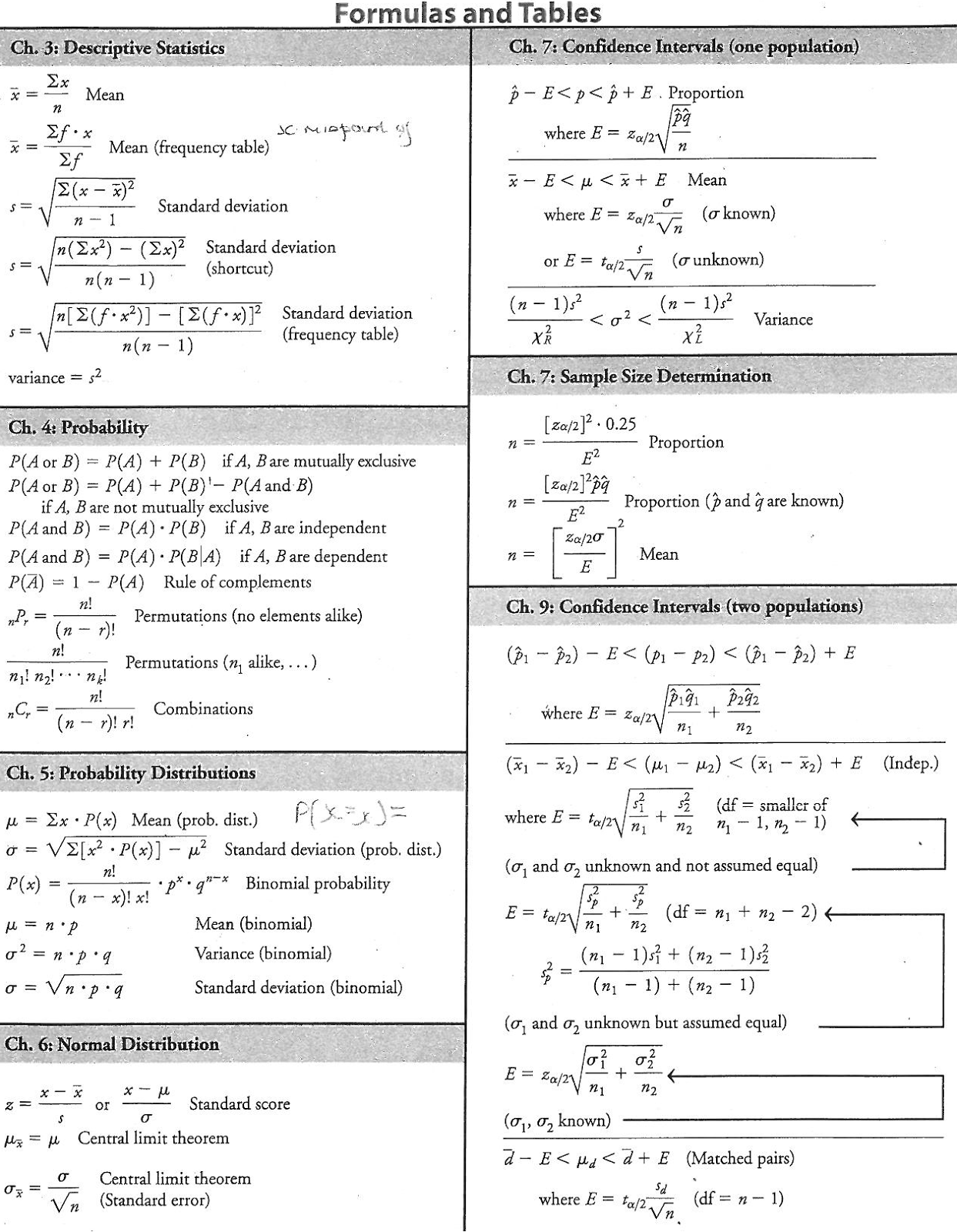
